3 Identification
In empirical research, identification refers to the process of establishing a clear and logical relationship between a cause and an effect. This involves demonstrating that the cause is responsible for the observed effect, and that there are no other factors that could potentially explain the effect. The goal of identification is to provide strong evidence that a particular factor is indeed the cause of a particular outcome, rather than simply coincidentally happen. In order to identify a cause-and-effect relationship, researchers can use experimental or non-experimental, that is, observational data, or both. Section 3.2 will discuss in greater detail how data can be collected that helps to evaluate causes and measure the magnitude of the effects of causes. Section 3.3.2 will explain some difficulties researchers must face when they aim to find empirical evidence on causal effects.
3.1 From anecdote to insight
Anecdotes are great. They are true stories—often intriguing, relatable, and easy to understand. They provide vivid examples that make abstract ideas more concrete and memorable. Whether it’s a personal experience or a captivating story about a successful business leader, anecdotes resonate because they tap into our natural affinity for storytelling. Their simplicity and emotional impact can make them powerful teaching tools.
And importantly, anecdotes are hard to contradict. Take, for example, the argument that smoking can’t be that harmful because your 88-year-old uncle has smoked his entire life and he is still in good health. It’s a tough claim to refute, as it’s a real-life example. However, the problem lies in extrapolating a single, isolated case to draw broader conclusions, which can be misleading.
However, while anecdotes can be persuasive, their strength is also their weakness. They represent isolated instances, and while it’s hard to deny the truth of an individual story, the danger lies in overgeneralizing from it. Anecdotes lack the rigorous analysis and breadth of evidence necessary to draw reliable conclusions. They don’t account for the full complexity of most situations, especially in business, where decisions are influenced by many interconnected factors.
In business, relying too heavily on anecdotes can lead to misguided conclusions. For example, a company might base its strategy on the success story of a famous entrepreneur without considering the countless failed ventures that didn’t make the headlines. This is known as survivorship bias, where the successes are visible, but the failures are hidden.
The challenge, then, is to take anecdotes and go beyond them. Instead of drawing direct conclusions, use them as starting points for deeper investigation. They can provide valuable hypotheses but need to be supported by data, rigorous analysis, and an understanding of the underlying principles at play. Anecdotes can inspire curiosity and point us in interesting directions, but they should be tested against a larger body of evidence to ensure that the insights we draw are reliable and applicable in a broader context.
Drawing insights from anecdotes is challenging, especially in business, for several reasons:
Limited sample size: Anecdotes are usually individual cases that do not reflect the full extent of a situation. In business, decisions often require data from large, diverse populations to ensure reliability. Relying on a single story or experience can lead to conclusions that are not universally valid.
Bias and subjectivity: Anecdotes are often influenced by personal perspectives, emotions or particular circumstances. Moreover, anecdotes often highlight success stories while ignoring failures. This is an example for the so-called Survivorship Bias.
Lack of context and the inability to generalize: Anecdotes often lack the broader context necessary to understand the underlying factors of a situation. Business problems tend to be complex and influenced by numerous variables such as market trends, consumer behavior and external economic conditions. Many of these variables change significantly over time. Without this context, an anecdote can oversimplify the problem and lead to incorrect decisions. Anecdotes are usually specific to a particular time, place or set of circumstances. They may not apply to different markets, industries or economic environments, which limits their usefulness for general decision-making. For example, learning only from the tremendous success of figures like Steve Jobs while ignoring the countless people who failed is like learning how to live a long life by talking to a single 90-year-old person. If that person happens to be obese and a heavy smoker, it doesn’t mean those behaviors contributed to their longevity.
Lack of data rigor: Anecdotes lack the rigor and precision of data-driven analysis where the empirical model that allows to identify causality and to measure the effect of causes is formally described.
Thus, to make informed business decisions, it is critical to base insights on systematic data analysis rather than anecdotal evidence, as anecdotes are too narrow, subjective and unreliable to guide complex business strategies.
3.2 Data acquisition
There are several ways to get data which allows you to (hopefully) identify a cause-and-effect relationship:
3.2.1 Interviews
An interview is normally a one-on-one verbal conversation. Interviews are conducted to learn about the participants’ experiences, perceptions, opinions, or motivations. The relationship between the interviewer and interviewee must be taken into account and other circumstances (place, time, face to face, email, etc.) should be taken into account. There are three types of interviews structured, semi-structured, and unstructured. Structured interviews use a set list of questions and hence are like a verbal surveys. In unstructured interviews the interviewer doesn’t use predetermined questions but only a list of topics to address. Semi-structured interviews are the middle ground. Semi-structured interviews require the interviewer to have a list of questions and topics pre-prepared, which can be asked in different ways with different interviewee/s. Semi-structured interviews increase the flexibility and the responsiveness of the interview while keeping the interview on track, increasing the reliability and credibility of the data. Semi-structured interviews are one of the most common interview techniques.
Structured interviews use a predetermined list of questions that must be asked in a specific order, improving the validity and trustworthiness of the data but lowering respondent response. Structured interviews resemble verbal questionnaires. In unstructured interviews, the interviewer has a planned list of subjects to cover but no predetermined interview questions. In exchange for less reliable data, this makes the interview more adaptable. Long-term field observation studies may employ unstructured interviews. The middle ground are interviews that are semi-structured. In semi-structured interviews, the interviewer must prepare a list of questions and themes that can be brought up in various ways with various interviewees.
Interviews allow you to address a cause-and-effect relationship fairly directly, and it can be a good idea to interview experts and ask some why and how questions to gather initial knowledge about a particular topic before further elaborating your research strategy. For example, I interviewed kindergarten teachers with many years of experience working with children, as well as other parents, to get information on how to solve the problem of my children throwing plates around the dining room. However, findings based on interviews are not very valid or reliable because the personal perceptions of both the interviewer and the interviewee can have an impact on the conclusions drawn. For example, I received very different tips and explanations because of the personal experiences of the people I interviewed. Unfortunately, I could not really ask my son why he was misbehaving. His vocabulary was too limited at the time, and even if he could speak, he would probably refuse to tell me the truth.
3.2.2 Surveys
In contrast to an interview a survey can be sent out to many different people. Surveys can be used to identify a cause-and-effect relationship by asking questions about both the cause and the effect and examining the responses. For example, if a researcher wanted to determine whether there is a relationship between a person’s level of education and their income, they could conduct a survey asking participants about their education level and their income. If the data shows that participants with higher levels of education tend to have higher incomes, it suggests that education may be a cause of higher income. However, it is important to note that surveys can only establish a correlation between variables, but it is difficult to claim that correlations that where found through the survey imply a causal relationship. To establish a causal relationship, a researcher would need to use other methods, such as an experiment, to control for other potential factors that might influence the relationship that the respondent does not see.
3.2.3 Case studies
Case studies involve in-depth examination of a single case or a small number of cases in order to understand a particular phenomenon. Case studies can be conducted using both quantitative and qualitative methods, depending on the research question and the data being analyzed. While it is reasonable to find causal effects in the particular case, it is problematic to generalize the causal relationship. To establish a general causal relationship, a researcher would need to use other methods, such as an experiment, to control for other potential factors that might influence the relationship that the respondent does not see.
3.2.4 Experiments
One way to clearly identify a cause-and-effect relationship is through experiments, which involve manipulating the cause (the independent variable) and measuring the effect (the dependent variable) under controlled conditions (we will later on define precisely what is meant here). Experiments can be conducted using both quantitative and qualitative methods. Here are some examples:
- A medical study in which a new drug is tested on a group of patients, while a control group receives a placebo.
- An educational study in which a group of students is taught a new method of learning, while a control group is taught using the traditional method.
- An agricultural study in which a group of crops is treated with a new fertilization method, while a control group is not treated.
- A study to determine the effect of a new training program on employee productivity might involve randomly assigning employees to either a control group that does not receive the training, or an experimental group that does receive the training. By comparing the productivity of the two groups, the researchers can determine if the new training program had a causal effect on employee productivity.
- A study to determine the effect of a new advertising campaign on sales might involve randomly assigning different groups of customers to be exposed to different versions of the campaign. By comparing the sales of the different groups, the researchers can determine if the advertising campaign had a causal effect on sales.
- In experimental economics, experimental methods are used to study economic questions. In a lab-like environment data are collected to investigate the size of certain effects, to test the validity of economic theories, to illuminate market mechanisms, or to examine the decision making of people. Economic experiments usually motivates and rewards subjects with money. The overall goal is to mimic real-world incentives and investigate things that cannot be captured or identified in the field.
- In behavioral economics, laboratory experiments are also used to study decisions of individuals or institutions and to test economic theory. However, it is done with a focus on cognitive, psychological, emotional, cultural, and social factors.
1 Source: https://commons.wikimedia.org/wiki/File:Daniel_Kahneman_(3283955327)_(cropped).jpg
In 2002 the Nobel Prize of Economics was awarded to Vernon L. Smith, I quote The Sveriges Riksbank Prize in Economic Sciences in Memory of Alfred Nobel (2002), “for having established laboratory experiments as a tool in empirical economic analysis, especially in the study of alternative market mechanisms” and Daniel Kahneman “for having integrated insights from psychological research into economic science, especially concerning human judgment and decision-making under uncertainty”.
The strength of evidence from a controlled experiment is generally considered to be strong. However, the external validity, i.e., the generalizability, should be considered as well. External validity is sometimes low because effects that you can identify and measure in a lab are sometimes only of minor importance in the field.
There are different types of experiments:
Randomized controlled trials (RCTs) are a specific type of an experiment that involve randomly assigning participants to different treatment groups and comparing the outcomes of those groups. RCTs are often considered the gold standard of experimental research because they provide a high degree of control over extraneous variables and are less prone to bias.
For a better explanation and some great insights into what an RCT actually is, please watch the video produced by UNICEFInnocenti and published on the YouTube channel of UNICEF’s dedicated research center, see https://youtu.be/Wy7qpJeozec and Figure 3.3.
2 Source: https://youtu.be/Wy7qpJeozec
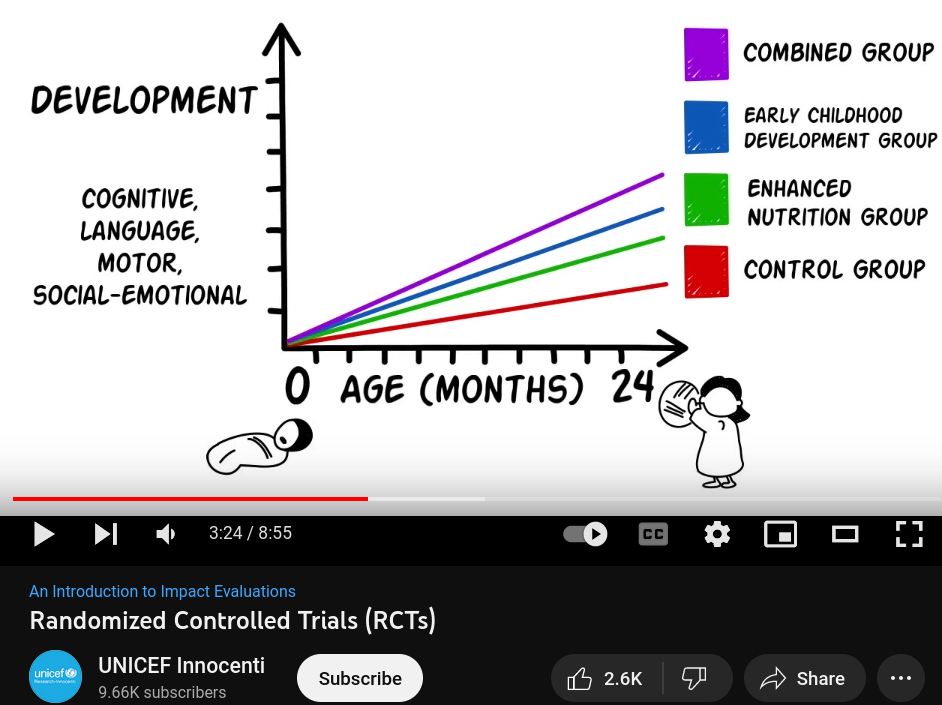
Quasi-experiments involve the manipulation of an independent variable, but do not involve random assignment of participants to treatment groups. Quasi-experiments are less controlled than RCTs, but can still provide valuable insights into cause-and-effect relationships.
Natural experiments involve the observation of naturally occurring events or situations that provide an opportunity to study cause-and-effect relationships. Natural experiments are often used when it is not possible or ethical to manipulate variables experimentally.
In a laboratory experiment, researchers manipulate an independent variable and measure the effect on a dependent variable in a controlled laboratory setting. This allows for greater control over extraneous variables, but the results may not generalize to real-world situations.
In a field experiment, researchers manipulate an independent variable and measure the effect on a dependent variable in a natural setting, rather than in a laboratory. This allows researchers to study real-world phenomena, but it can be more difficult to control for extraneous variables.
3.2.5 Observational data
3 Source: https://pixabay.com/images/id-5029286/
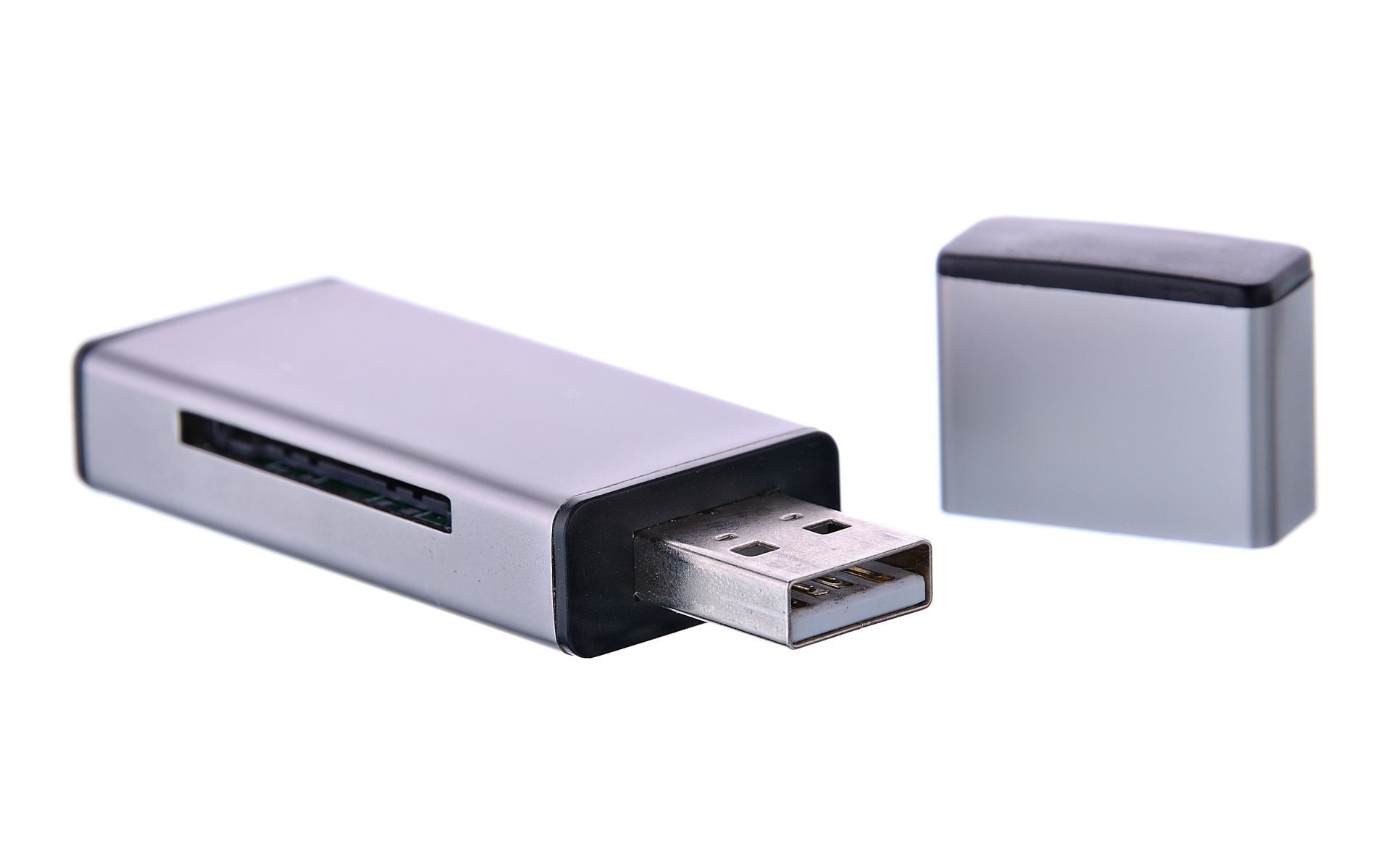
Observational data are data that had been observed before the research question was asked or being collected independently from the study. To understand how observational data can be used to constitute a causal relationship is a bit tricky because there is only one world and only one reality at a time. In other words, we usually miss a counterfactual which we can use for a comparison. Take, for example, the past COVID-19 pandemic, where you chose to be vaccinated or not. Regardless of what you chose, we will never find out what would have happened to you if you had chosen differently. Maybe you would have died, maybe you would have gotten more or less sick, or maybe you wouldn’t have gotten sick at all. We don’t know, and it’s impossible to find out because it’s impossible to observe the counterfactual outcomes. This makes it difficult to establish causality from observational data. However, ingenious minds have found reasonable procedures and methods to extract some level of knowledge from observational data that allows us to infer causal relationships from observational data where we cannot directly observe the counterfactual outcome. We will come back to these methods later on.
In the upcoming sections, however, we will discuss experimental research designs including randomized controlled trials (RCTs) which are considered to be the “gold standard for measuring the effect of an action” (Taddy, 2019, p. 128). RCTs can be used, for example, to study the effectiveness of drugs by observing people randomly assigned to three groups, one taking the pill (or treatment), a second receiving a placebo, and a third taking nothing. If the first group responds in any way differently than the other groups, the drug has an effect. Before explaining an RCT in more detail, we need to be clear about the fundamental problem of causal inference. This will be discussed in the following.
3.3 Causal inference
4 Source: Cunningham (2021)
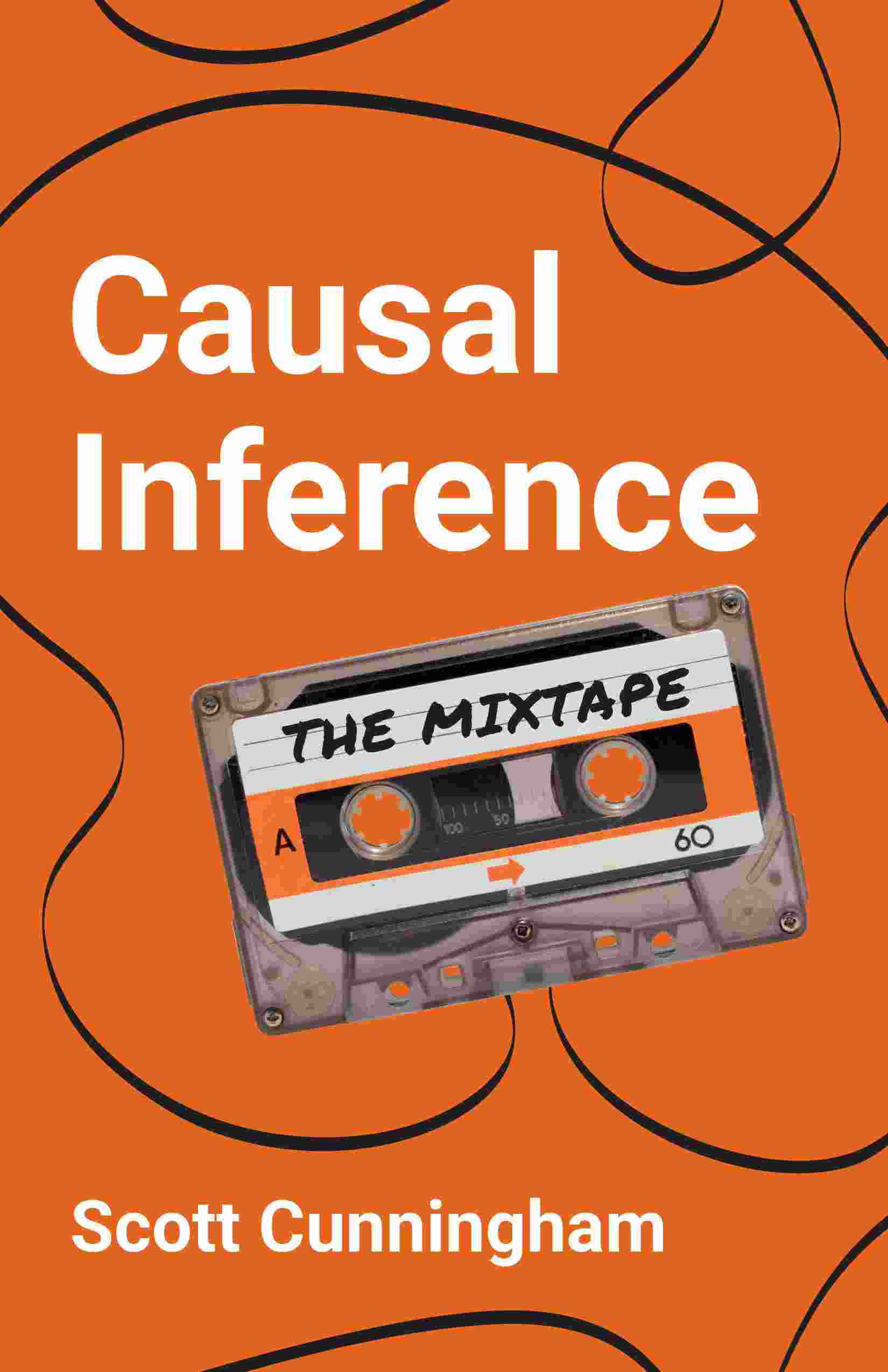
As Cunningham (2021) explains in his book (see Figure 3.5), establishing causality is very challenging. Causal inference can assist to some extent. It is the process of establishing causal relationships between variables, aiming to determine whether a change in one variable (the cause or independent variable) leads to a change in another variable (the effect or dependent variable). This process goes beyond mere association or correlation and seeks to establish that one event or factor directly influences another. Various methods of causal inference exist, and this section along with the upcoming chapters will discuss these methods. All methods share a common goal: identifying and measuring a relationship without any bias.
3.3.1 The fundamental problem of causal inference
Unfortunately, claiming a causal relationship to be empirically true is often not straightforward. The main reason for this lies in the so-called fundamental problem of causal inference, which is the issue of observing only one of the potential outcomes for each unit in a study. This means we lack the counterfactual outcome, which is the hypothetical outcome that would have occurred if a subject or unit had experienced a different condition or treatment than what actually happened. Thus, the fundamental problem of causal inference is actually a missing data problem.
For example, consider my son, who enjoyed throwing plates from the table. He must decide between throwing a plate or not, but he cannot do both simultaneously – an ability only possible in fictional movies like “Everything Everywhere All at Once”. Of course, my son can conduct an experiment by throwing a plate now and later deciding not to throw a plate. After observing both actions, he may claim to have found evidence that throwing a plate causes noise. However, he can never be 100% certain that the noise he heard after throwing the plate was solely caused by his action. It could be a coincidence that something else caused the noise at precisely the same time, like one of his siblings throwing a fork. He merely assumes it was due to his action. To be more certain, he might repeat the experiment hundreds of times. Even then, he can never be 100% sure. It is still not proof in a logical sense because an external factor could theoretically cause the noise. However, this is where statistics come into play: nowing the environment and the setup of his actions, it becomes extremely unlikely that the noise was not caused by his action. Knowing the setup means, we now that hasn’t been any external factor that may have caused a false a causal fallacy. As Scott Cunningham emphasizes, “prior knowledge is required in order to justify any claim about a causal finding”:
Cunningham (2021, ch. 1.3): “It is my firm belief, which I will emphasize over and over in this book, that without prior knowledge, estimated causal effects are rarely, if ever, believable. Prior knowledge is required in order to justify any claim of a causal finding. And economic theory also highlights why causal inference is necessarily a thorny task.”
To illustrate that the fundamental problem of causal inference is actually a missing data problem, let’s consider the fictitious example data presented in Table 3.1. For different individuals, dentoted as \(i\), we know whether they were received treatment \((T=1)\) or did not receive treatment \((T=0)\), as well as whether the outcome was positive \((Y=1)\) or negative \((Y=0)\). Since we do not observe the counterfactual outcomes, we are unable to determine the individual treatment effect (ITE), which is expressed as \(Y_i(1)-Y_i(0)\).
\(i\) | \(T\) | \(Y\) | \(Y_i(1)\) | \(Y_i(0)\) | \(Y_i(1)-Y_i(0)\) |
---|---|---|---|---|---|
1 | 0 | 0 | ? | 0 | ? |
2 | 1 | 1 | 1 | ? | ? |
3 | 1 | 0 | 0 | ? | ? |
4 | 0 | 0 | ? | 0 | ? |
5 | 0 | 1 | ? | 1 | ? |
3.3.2 Correlation does not imply causation
Correlation refers to a statistical relationship between two variables, where one variable tends to increase or decrease as the other variable also increases or decreases. However, just because two variables are correlated does not necessarily mean that one variable causes the other. This is known as the correlation does not imply causation principle.
For example, across many areas the number of storks is correlated with the birth rate of babies (see Matthews, 2000). However, this does not mean that the presence of storks causes an increase in the birth rate. It is possible that both the number of storks and the number of babies born are influenced by other factors, such as the overall population density or economic conditions in the area.
Therefore, it is important to carefully consider all possible explanations (confounders) for a correlation and to use data to disentangle the true cause-and-effect relationship between variables.
5 Source: https://youtu.be/DFPm_a-_uJM
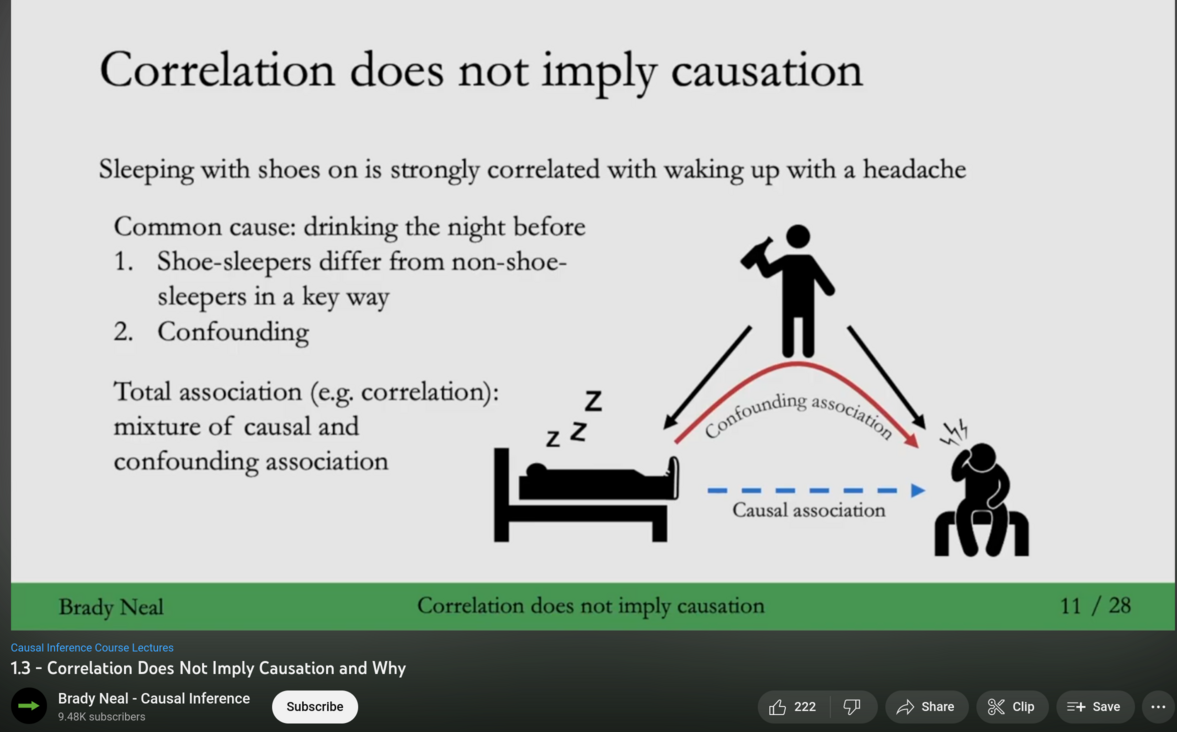
Watch the video of Brady Neal’s lecture Correlation Does Not Imply Causation and Why. Alternatively, you can read chapter 1.3 of his lecture notes (Neal, 2020) which you find here.
3.3.3 Simpsons Paradox
6 Source: The photography is public domain and stems from the Library of Congress Prints and Photographs Division Washington, see: http://hdl.loc.gov/loc.pnp/pp.print.
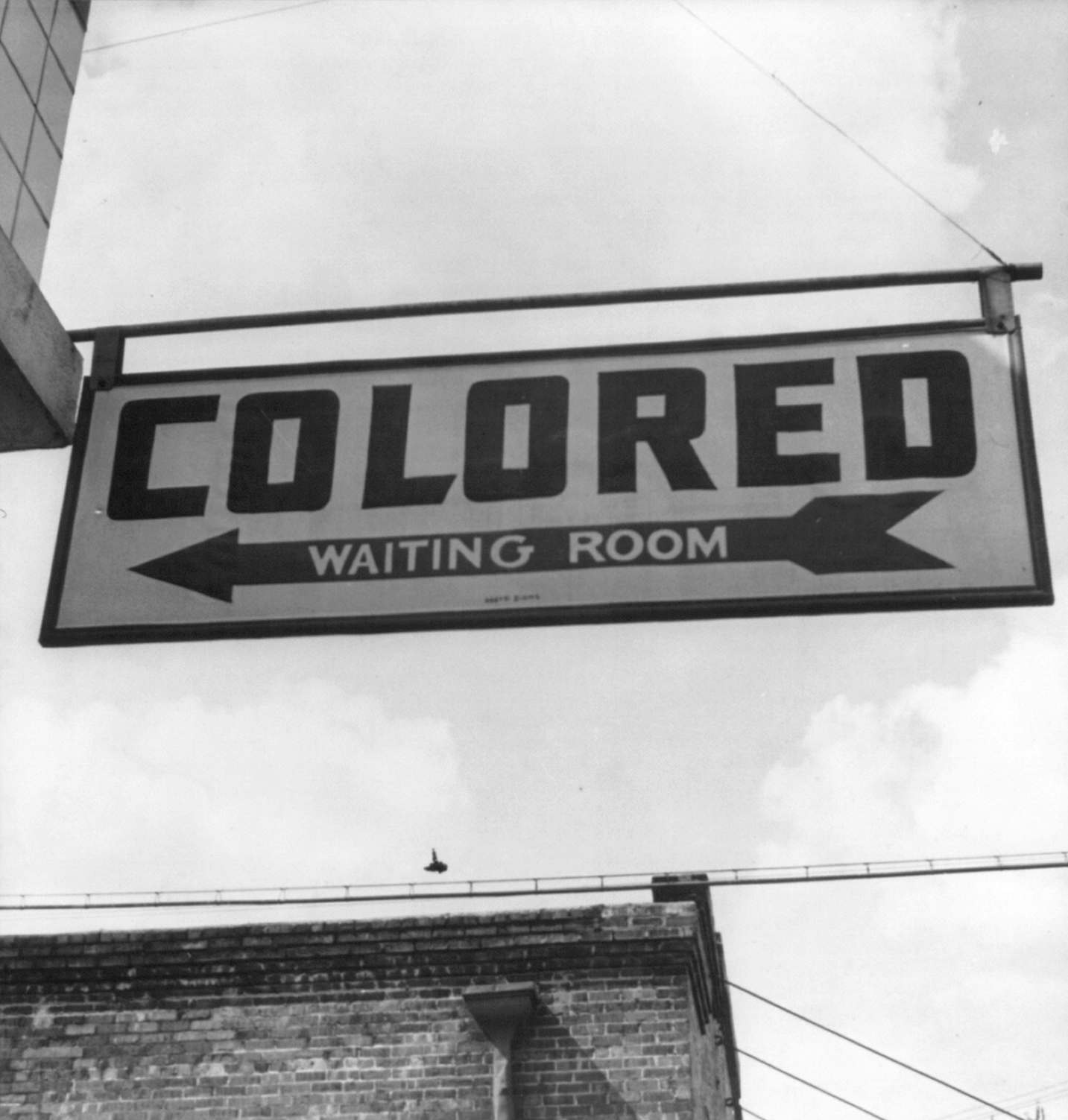
Discrimination is bad. Whenever we see it, we should try to find ways to overcome it. De jure segregation mandated the separation of races by law is clearly discriminatory. Other forms of discrimination, however, are often more difficult to spot and as long we don’t have good evidence for discrimination, we should not judge prematurely. That means, we should be sure that we see an act of making unjustified distinctions between individuals based on some categories to which they belong or perceived to belong. For example, if men and women are treated differently without an acceptable reason, we consider it discriminative.
However, as the following example discussed in Bickel et al. (1975) will show, it is often challenging to identify discrimination. In 1973, UC Berkeley was accused of discrimination because it admitted only 35% of female applicants but 44% of male applicants overall. The difference was statistical significant and based on that many people protested claiming justice and equality. However, it turned out that the selection of students was not discriminative against women but against men. Accordingly to Bickel et al. (1975) the different overall admission rates can be largely explained by a “tendency of women to apply to graduate departments that are more difficult for applicants of either sex to enter” (Bickel et al., 1975, p. 403). Figure Figure 3.8 taken from Bickel et al. (1975, p. 403) visualizes this fact. Looking on the decisions within the departments seperately, there is even a “statistically significant bias in favor of women” (Bickel et al., 1975, p. 403).
7 Source: Bickel et al. (1975, p. 403)
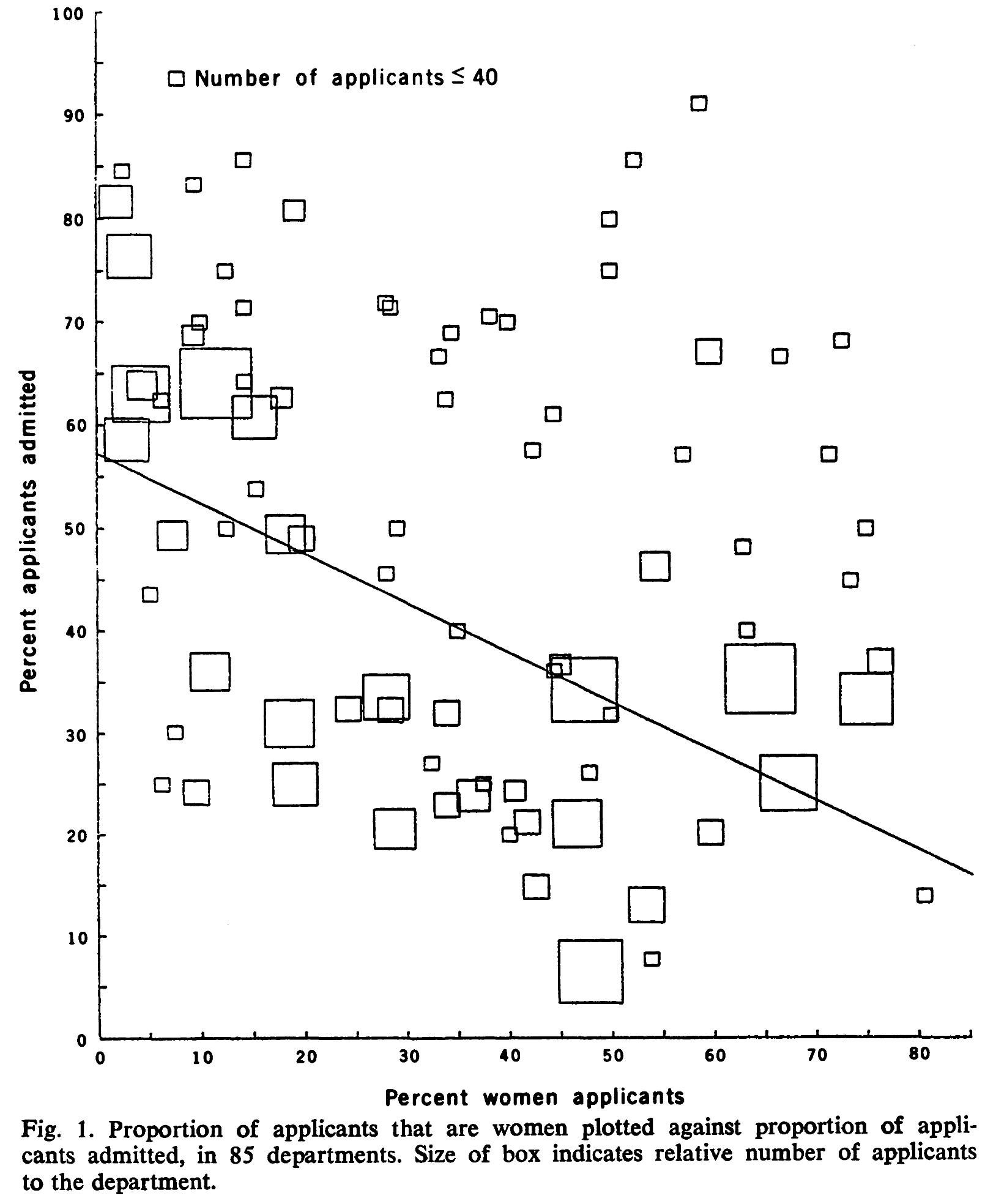
Here is a summary of Bickel et al. (1975, p. 403):
“Examination of aggregate data on graduate admissions to the University of California, Berkeley, for fall 1973 shows a clear but misleading pattern of bias against female applicants. Examination of the disaggregated data reveals few decision-making units that show statistically significant departures from expected frequencies of female admissions, and about as many units appear to favor women as to favor men. If the data are properly pooled, taking into account the autonomy of departmental decision making, thus correcting for the tendency of women to apply to graduate departments that are more difficult for applicants of either sex to enter, there is a small but statistically significant bias in favor of women. The graduate departments that are easier to enter tend to be those that require more mathematics in the undergraduate preparatory curriculum. The bias in the aggregated data stems not from any pattern of discrimination on the part of admissions committees, which seem quite fair on the whole, but apparently from prior screening at earlier levels of the educational system. Women are shunted by their socialization and education toward fields of graduate study that are generally more crowded, less productive of completed degrees, and less well funded, and that frequently offer poorer professional employment prospects.”
3.3.4 Rubin causal model
If we are interested in the causal effect of a certain treatment on an outcome, we need to compare the outcome, \(Y\), of an individuals, \(i\), who received the treatment, \(1\), to the outcome, \(Y\), of the same individual, \(i\), who did not receive the treatment, \(0\):
\[ ITE_i=Y_i(1)-Y_i(0). \]
Unfortunately, as discussed in Section 3.3.1, this individual treatment effect (ITE) does not exist as person \(i\) can either be treated or not, but not both simultaneously. Since the counterfactual outcome is missing for each individual, we cannot observe the actual causal effect.
The Rubin model, also known as the potential outcomes framework, provides a theoretical framework for identifying causality in the context of missing data-existing.
In the model, each subject, denoted with \(i\) (for example, a person, a school), has two potential outcomes: one outcome if the subject receives the treatment (treatment condition denoted with \(T=1\)) and another outcome if the does not receive the treatment (control condition denoted with \(T=0\)). In short, the model specifies that you can use the difference of the average of a group that received the treatment and the average of the group that did not received the treatment and use it as a substitute for the ITE: \[ \mathbb{E} [\underbrace{Y_i(1)-Y_i(0)}_{ITE}] = \underbrace{\mathbb{E}[Y(1)] - \mathbb{E}[Y(0)]}_{ATE}. \tag{3.1}\]
However, the ATE is only equal to the expected ITE if certain assumptions are fulfilled. The upcoming sections will discuss these assumptions.
3.3.5 Its difficult to overcome the fundamental problem
Keele (2015, p. 314): “An identification analysis identifies the assumptions needed for statistical estimates to be given a causal interpretation.”
In the following we will discuss conditions that need to hold in order to empirically draw causal conclusions from the ATE without bias. This is important because equation Equation 3.1 does not necessarily hold when using observational data without a more elaborated identification strategy.
3.3.5.1 Example
Suppose we want to measure the effect of a vaccine on survival rates. We observed the residents of a small city with 2,000 inhabitants over the course of 30 days. On day 1, we arrived in town and injected the vaccine to 200 individuals. By day 30, we counted the deceased in both groups: four died in the vaccinated group, while eighteen died in the group of 1,800 unvaccinated individuals. With a survival rate of 98% in the vaccinated group and 99% in the unvaccinated group, it may appear that the vaccine lowers the survival rate. Imagine that study is real, would you claim that the vaccine kills because according to Equation 3.1 we could use the ATE to indicate the ITE?
The answer is yes, but only if the assumptions of ignorability (Section 3.3.5.2) and unconfoundedness (Section 3.3.5.3) are satisfied.
In brief, ignorability means that the 200 treated individuals are not systematically different from the other 1,800 individuals regarding characteristics that have an impact of the chances to survive. Considering the fact that we cannot randomly select 200 individuals from the 2,000 inhabitants due to legal constraints (as everyone has the right to choose whether or not to receive the vaccine), we must consider who is willing to get vaccinated. This selection bias may pose issues, as vulnerable populations often have a higher willingness to accept the vaccine compared to younger and healthier individuals who may fear the disease less. For example, if we vaccinated individuals with preexisting conditions that make them more vulnerable, such as the elderly or those with chronic illnesses, we cannot assume that the ATE is equal to the ITE. This is because the overall mortality risk is higher among those who received the vaccine.
Unconfoundedness means that there are no other factors that could explain both the likelihood of receiving the vaccine and the likelihood of death. For example, if vaccinated individuals were not required to stay at home during these 30 days, their likelihood of dying may increase due to greater exposure to risky situations and other people, which in turn raises their chances of contracting a disease.
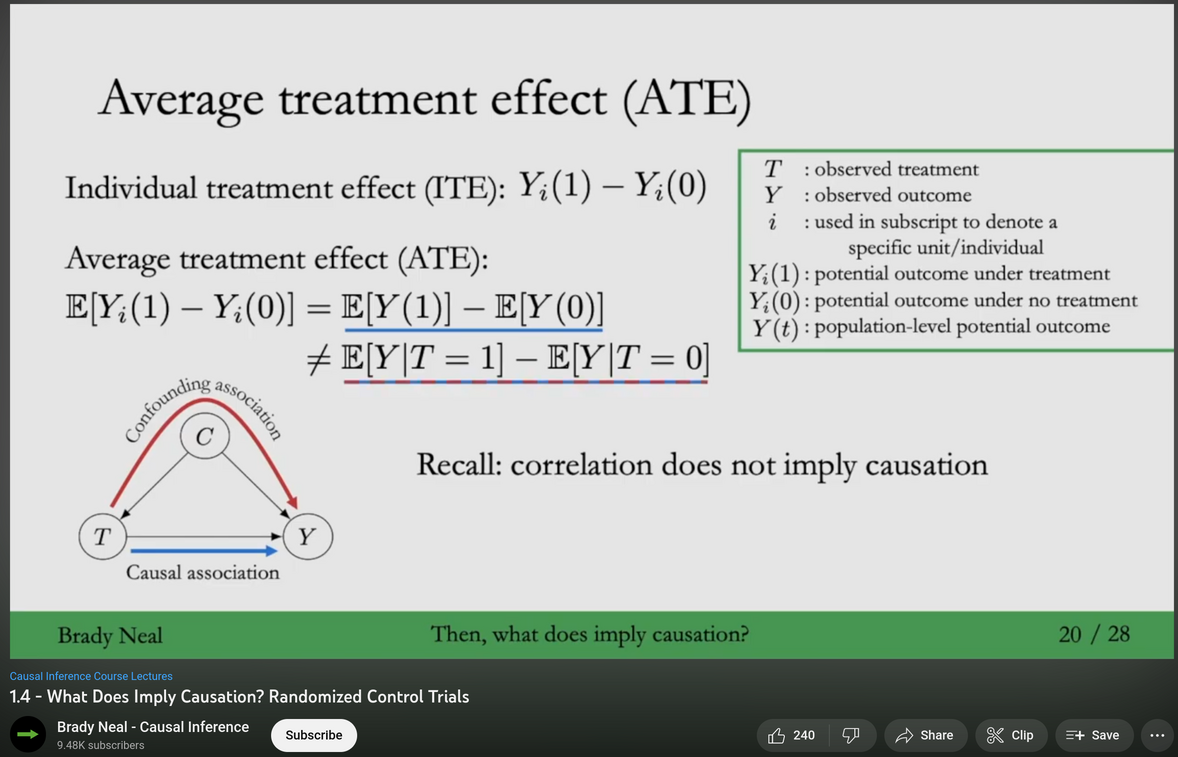
Watch the video of Brady Neal’s lecture What Does Imply Causation? Randomized Control Trials (see Figure 3.9). Alternatively, you can read Neal (2020, ch. 2) of his lecture notes, see here.
3.3.5.2 Ignorability
Referring to table Table 3.1, Brady Neal (2020) wrote:
“what makes it valid to calculate the ATE by taking the average of the Y(0) column, ignoring the question marks, and subtracting that from the average of the Y(1) column, ignoring the question marks?” This ignoring of the question marks (missing data) is known as ignorability. Assuming ignorability is like ignoring how people ended up selecting the treatment they selected and just assuming they were randomly assigned their treatment” (Neal, 2020, p. 9)
Ignorability means that the way individuals are assigned to treatment and control groups is irrelevant for the data analysis. Thus, when we aim to explain a certain outcome, we can ignore how an individual made it into the treated or control group. It has also been called unconfoundedness or no omitted variable bias. We will come back to these two terms in Section 3.4 and in Chapter 5.
Randomized controlled trials (RCTs) are characterized by randomly assigning individuals to different treatment groups and comparing the outcomes of those groups. Thus, RCTs are essentially build on the assumption of ignorability which can be written formally like \[ (Y(1), Y(0)) \perp T. \]
This notation indicates that the potential outcomes of an individual, \(Y\), are independent of whether they have actually received the treatment. The symbol “\(\perp\)” denotes independence, suggesting that the outcomes \(Y(1)\) and \(Y(0)\) are orthogonal to the treatment \(T\).
The assumption of ignorability allows to write the ATE as follows: \[\begin{align} \mathbb{E}[Y(1)]-\mathbb{E}[Y(0)] & =\mathbb{E}[Y(1) \mid T=1]-\mathbb{E}[Y(0) \mid T=0] \\ & =\mathbb{E}[Y \mid T=1]-\mathbb{E}[Y \mid T=0]. \end{align}\]
Another perspective on this assumption is the concept of exchangeability. Exchangeability refers to the idea that the treatment groups can be interchanged such that if they were switched, the new treatment group would have the same outcomes as the old treatment group, and the new control group would have the same outcomes as the old control group.
3.3.5.3 Unconfoundedness
While randomized controlled trials (RCTs) assume the concept of ignoreability, most observational data present challenges in drawing causal conclusions due to the presence of confounding factors that affect both (1) the likelihood of individuals being part of the treatment group and (2) the observed outcome. For example, regional factors can affect both the number of storks and the number of babies born in a region. These factors are typically referred to as confounders, which we discussed in Section 3.3.2 as having the potential to create the illusion of a causal impact where none exists. However, empirical methods are available to control for these confounders and prevent the violation of the ignoreability assumption. Formally, the assumption can be written as \[ (Y(1), Y(0)) \perp T \mid X. \] This allows to write the ATE as follows: \[\begin{align} \mathbb{E}[Y(1)\mid X]-\mathbb{E}[Y(0)\mid X] & =\mathbb{E}[Y(1) \mid T=1, X]-\mathbb{E}[Y(0) \mid T=0, X] \\ & =\mathbb{E}[Y \mid T=1, X]-\mathbb{E}[Y \mid T=0, X]. \end{align}\]
This means that we need to control for all factors (X) that influence both groups. We will revisit this topic in Section 3.4, where we will discuss the various functional impacts that must be considered to avoid causal bias.
3.4 Statistical control requires causal justification
Scientific research revolves around challenging our own views and findings. A good researcher does not merely present their results; instead, they engage in discussions about potential limitations and pitfalls to draw valid conclusions. Engaging in polemics goes against the essence of good research. We should not conceal potential weaknesses in our scientific strategy or empirical approach; rather, we should emphasize their existence. Even if this disappoints individuals seeking easy answers, it is crucial to acknowledge these limitations. The Catalogue of Bias is an excellent resource that provides insight into various potential pitfalls and challenges encountered during research, which may sometimes be difficult to completely rule out.
Solutions to the exercises
8 A VAR is a statistical model used to capture the relationship between multiple quantities as they change over time.